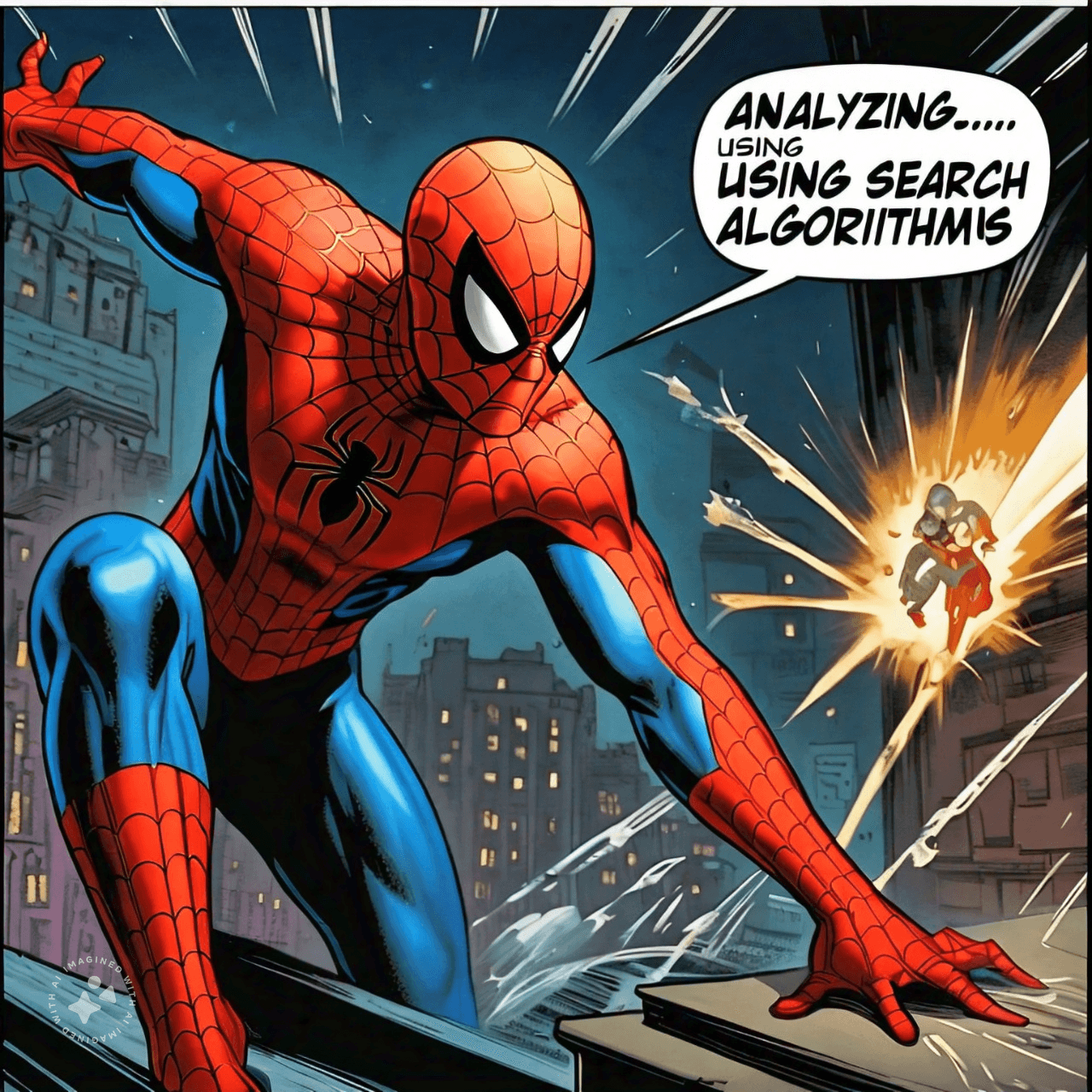
Searching Algorithms - All you need to know
- Searching Algorithms
- Linear Search
- Binary Search
- Depth First Search (DFS)
- Breadth First Search (BFS)
- Amortization of Searching Algorithms
- Comparison of Linear Search, Binary Search, DFS, and BFS
- Time Complexities
- Space Complexities
- Amortized Analysis
- Linear and Binary Search
- DFS and BFS
- Key Differences
Searching Algorithms
Linear Search
linear_search.py
def linear_search(arr, target):
for i in range(len(arr)):
if arr[i] == target:
return i
return -1
# Time Complexity: O(n)
# Space Complexity: O(1)
Binary Search
binary_search.py
def binary_search(arr, target):
left, right = 0, len(arr) - 1
while left <= right:
mid = (left + right) // 2 #OR left + (right - left) // 2 for large numbers
if arr[mid] == target:
return mid
elif arr[mid] < target:
left = mid + 1
else:
right = mid - 1
return -1
# Time Complexity: O(log n)
# Space Complexity: O(1)
Depth First Search (DFS)
dfs.py
def dfs(graph, start, visited=set()):
if start not in visited:
print(start)
visited.add(start)
for neighbor in graph[start]:
dfs(graph, neighbor, visited)
if __name__ == "__main__":
graph = {
'A': ['B', 'C'],
'B': ['D', 'E'],
'C': ['F'],
'D': [],
}
dfs(graph, 'A')
# Time Complexity: O(V + E)
# Space Complexity: O(V)
Breadth First Search (BFS)
bfs.py
def bfs(graph, start):
visited = set()
queue = deque([start])
while queue:
vertex = queue.popleft()
if vertex not in visited:
print(vertex)
visited.add(vertex)
queue.extend(graph[vertex] - visited)
if __name__ == "__main__":
graph = {
'A': ['B', 'C'],
'B': ['D', 'E'],
'C': ['F'],
'D': [],
}
bfs(graph, 'A')
# Time Complexity: O(V + E)
# Space Complexity: O(V)
Amortization of Searching Algorithms
Comparison of Linear Search, Binary Search, DFS, and BFS
Time Complexities
Algorithm | Average Case | Best Case | Worst Case |
---|---|---|---|
Linear Search | O(n) | O(1) | O(n) |
Binary Search | O(log n) | O(1) | O(log n) |
DFS | O(V + E) | O(V + E) | O(V + E) |
BFS | O(V + E) | O(V + E) | O(V + E) |
Space Complexities
Algorithm | Average Case | Best Case | Worst Case |
---|---|---|---|
Linear Search | O(1) | O(1) | O(1) |
Binary Search | O(1) | O(1) | O(1) |
DFS | O(h) | O(h) | O(V) |
BFS | O(w) | O(1) | O(V) |
Where V is the number of vertices, E is the number of edges, h is the height of the tree (for DFS), and w is the maximum width of the tree (for BFS).
Amortized Analysis
Linear and Binary Search
Linear and binary search algorithms have consistent performance across operations, so their amortized analysis is the same as their worst-case analysis.
DFS and BFS
For DFS and BFS, the amortized analysis is particularly relevant when considering a sequence of operations on a graph or tree structure[1].
DFS (Depth-First Search):
- DFS typically uses a stack (either explicitly or via recursion)
- Amortized time complexity: O(V + E)
- Each vertex is pushed and popped at most once, leading to an amortized cost of O(1) per vertex[4]
BFS (Breadth-First Search):
- BFS uses a queue for its implementation
- Amortized time complexity: O(V + E)
- Each vertex is enqueued and dequeued at most once, also resulting in an amortized cost of O(1) per vertex[4]
Key Differences
Exploration Strategy:
- DFS explores as far as possible along each branch before backtracking
- BFS explores all neighbors at the present depth before moving to the next level[1]
Data Structures:
- DFS uses a stack or recursion
- BFS uses a queue[1]
Applicability:
- DFS is better for problems where you need to explore all paths
- BFS guarantees shortest paths in unweighted graphs[1]
Space Efficiency:
- DFS can be more space-efficient for deep graphs
- BFS can be more space-efficient for wide, shallow graphs
Completeness:
- BFS is complete and will find a solution if one exists
- DFS may not terminate on infinite graphs